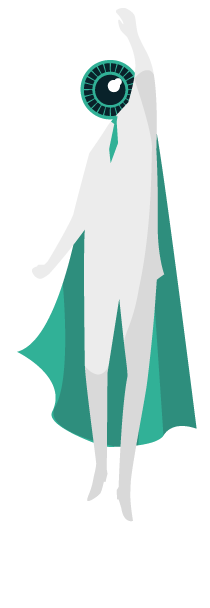
How did red dot® perform at Somerset NHS Foundation Trust?
3,794
images processed in just 11 hours
15.7
seconds on average per scan
15%
saving in radiologist workload
57%
reduction in waiting times for suspected lung cancer patients
Of the 3,794 images processed during the trial, the model classified 147 (3.9%) exams as SLC, and 562 (14.8%) as HCN. On average, the red dot® algorithm returned a result in 15.7 seconds.
Read the full case study:
What is red dot CXR?
The red dot® chest x-ray (CXR) solution developed by Behold.ai is a CE/UKCA certified artificial intelligence (AI) model that helps radiology departments fast-track the diagnosis of patients with suspected lung cancer and rule-out normal CXR exams. It does so by prioritising exams classified as suspected lung cancer (SLC) for reporting, and by auto-reporting exams classified as high confidence normal (HCN) and removing these from radiologists’ worklists.
In more detail, the red dot® algorithm classifies exams as SLC when it identifies CXR features that may indicate the presence of lung cancer; this can consist of a lung nodule, a lung mass, hilar enlargement or a combination of these. The red dot® platform is also capable of providing a heatmap that highlights identified suspicious areas on CXRs, thus helping explain the algorithm’s decision to radiologists. An example of a CXR classified as SLC and its associated heatmap can be seen here.
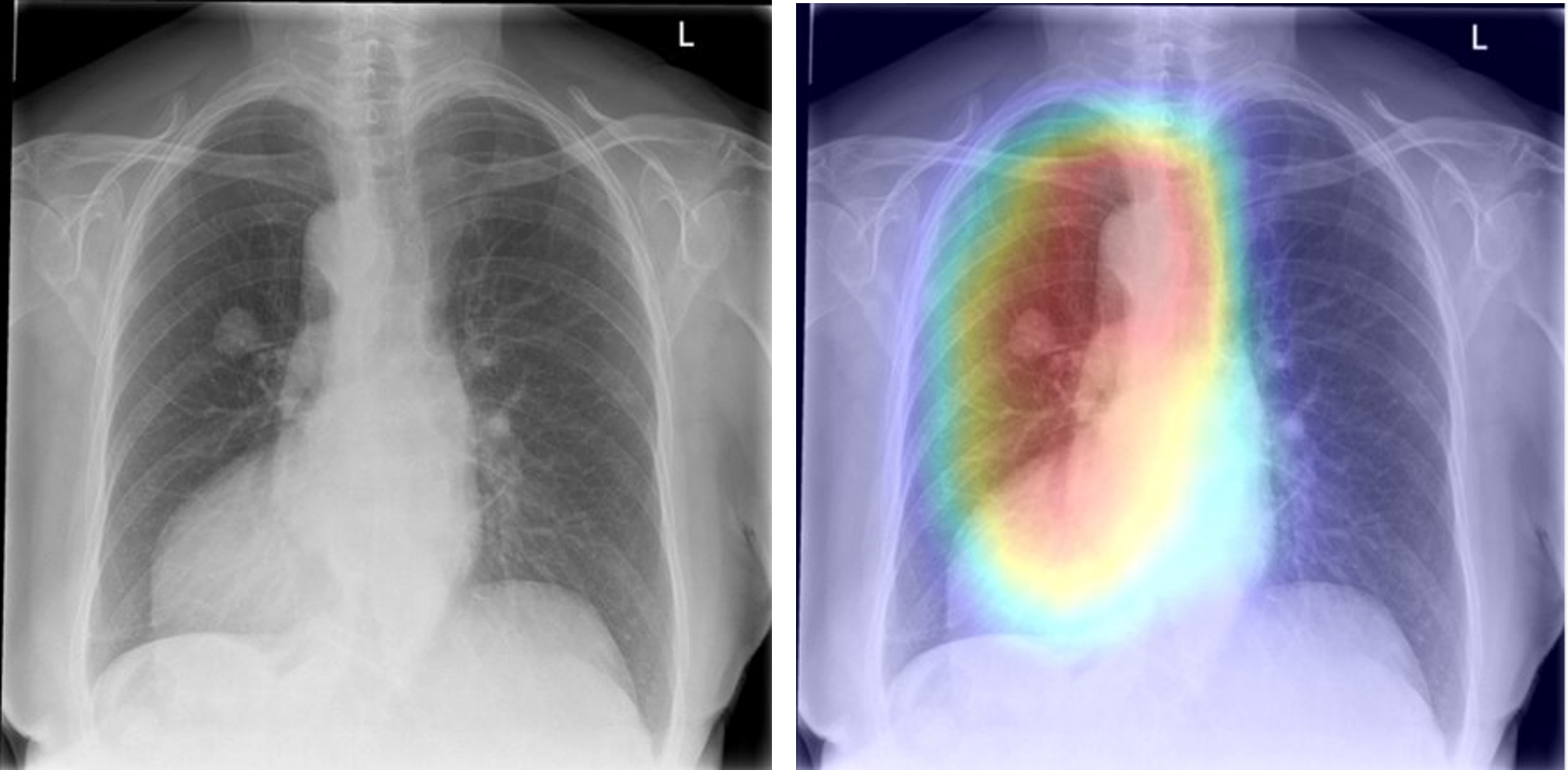
In turn, the red dot® algorithm classifies exams as HCN when it does not identify any abnormalities, which allows it to indicate with high confidence that an exam is normal. By auto-reporting HCN exams and removing them from worklists, the HCN functionality can reduce workload pressures and allow radiologists to better focus on more complex and urgent cases. As per Behold.ai’s CQC registration, all auto-reported exams are audited within 24 hours by a senior consultant FRCR radiologist.
In retrospective studies, the red dot® CXR algorithm has shown that it can classify 15% of CXRs with an error rate of just 0.33%, and that it can improve and standardise radiologist performance for identifying early-stage lung cancer [1].
How did the trial work?
The Somerset NHS Foundation Trust deployed the red dot®CXR solution for a three-month pilot period to evaluate the use of the algorithm in supporting the Trust meet its national targets for lung cancer diagnosis.
To monitor the accuracy of the red dot®CXR algorithm, radiologists indicated whether they agreed or disagreed with the algorithm’s classification in their exam text reports. All exams reported as disagreements were reviewed and arbitrated by experienced radiologists. To maximize patient safety and performance standards throughout the trial, all exams classified as HCN by the red dot®CXR solution were still reported by a radiologist.
What were the results?
Of the 3,794 images processed during the trial, the model classified 147 (3.9%) exams as SLC, and 562 (14.8%) as HCN. On average, the red dot® algorithm returned a result in 15.7 seconds.
Within this trial, Behold.ai demonstrated a notable reduction in the time taken to progress patients through the lung cancer pathway, and it showed that the real performance of our algorithm closely matches that of our peer-reviewed published results [1]. In more detail, the red dot® algorithm demonstrated time-saving benefits for the SomersetNHS Foundation Trust that can promote faster diagnosis for patients, as the deployment of the algorithm more than halved the time between initial X-ray screening and a CT scan (the gold standard for detecting lung cancer), from 7 days to 3 days.
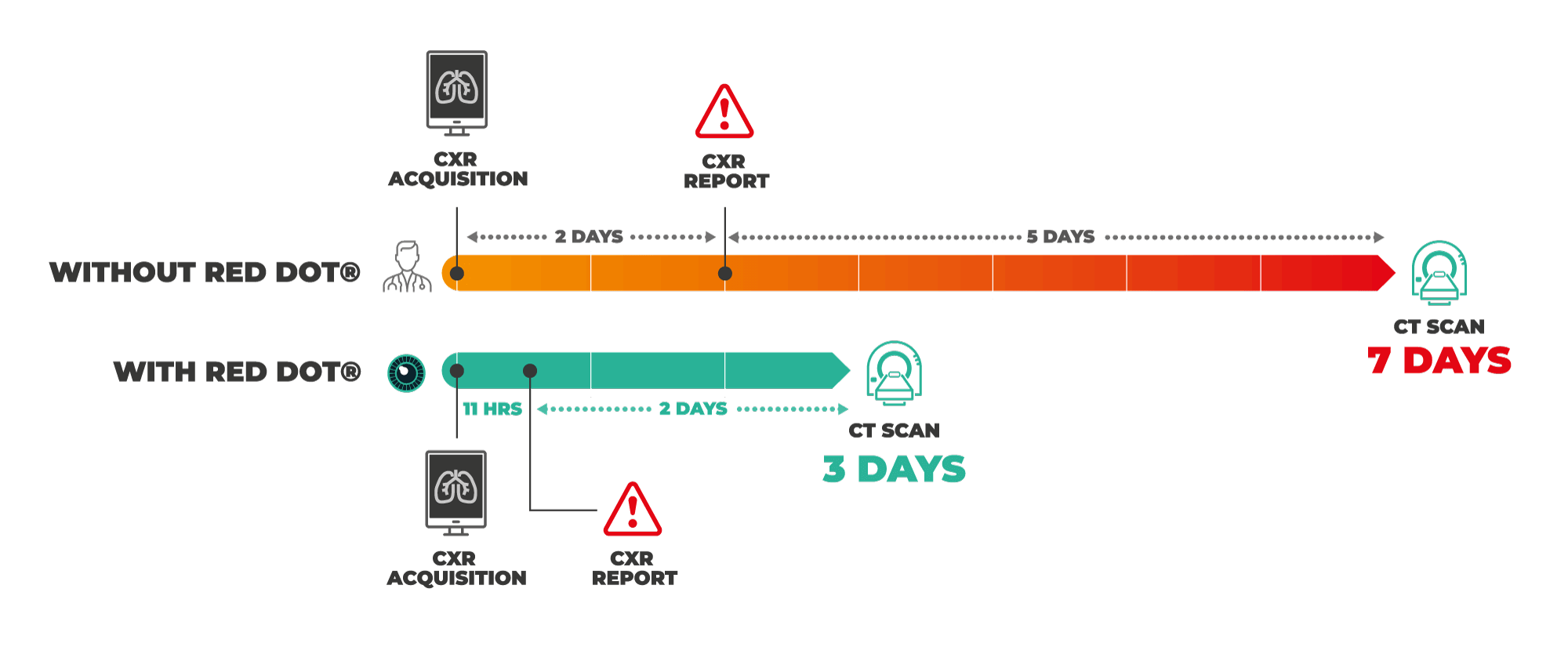
Accelerating lung cancer diagnosis
The trial demonstrated the effectiveness of red dot® platform for flagging exams as SLC. As previously mentioned, the model flagged 147 (3.9%) exams as Suspected Lung Cancer (SLC), 19 of which (12.9%) were later diagnosed with cancer. Of the exams that were classed as SLC by the model, 75% were reported within 6 hours by the Trust. These figures indicate that scans with suspected lung cancer are being effectively prioritised for reporting and fast-tracking diagnosis.
Reducing workload with rule-out Normal
The algorithm ruled out 14.8% of total scans as High Confidence Normal (HCN), effectively indicating that they can be removed from radiologists’ workload and auto-reported. It did so with a high degree of accuracy; the model performed with a negative predictive value (NPV) of 97.7% and a false negative rate of 0.34%. This saves time and resources by easing the burden on radiologists and allowing them to prioritise their workload. The few discrepancies (13 out of 562 cases) that were found between hospital radiologists and the model’s HCN classification were arbitrated by two independent hospital radiologists, and none of these discrepancy cases were found to have clinically significant implications.
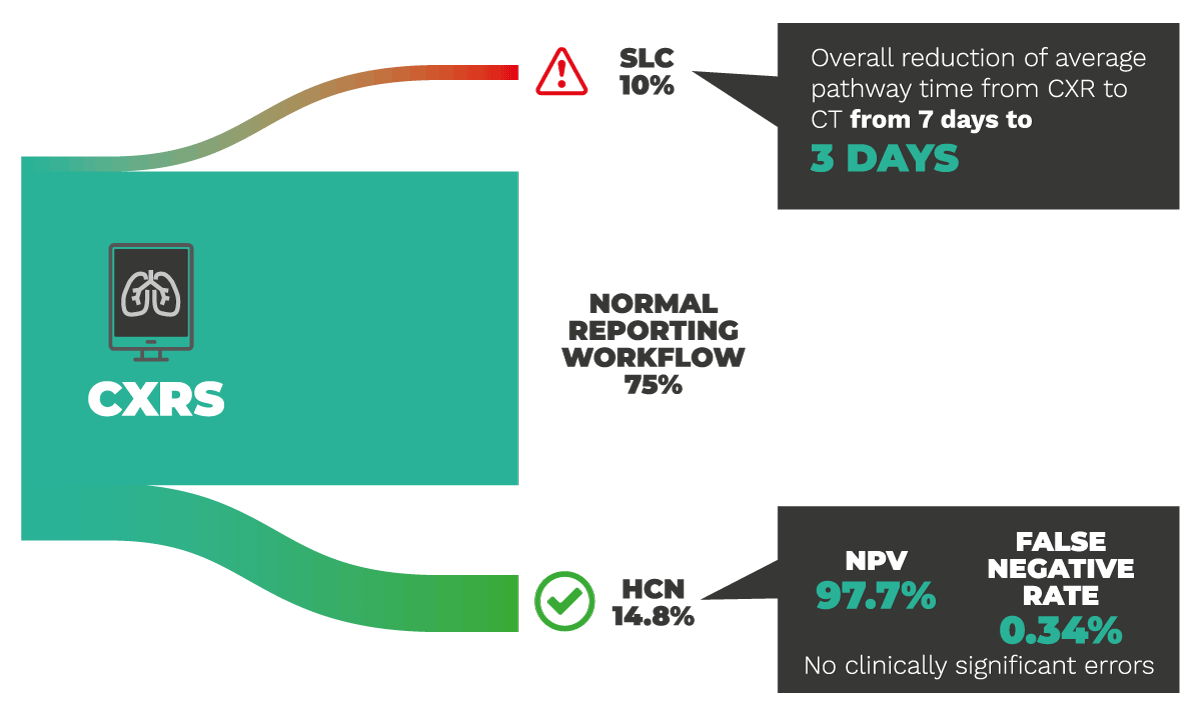
Conclusions and next steps
This trial demonstrated that Behold.ai’s red dot® CXR solution has a robust real-world performance, and that its use can provide significant time-saving benefits in the lung cancer pathway while reducing radiologist workloads by 15% with no significant misses.
The study was supported by funding from Somerset, Wiltshire Avon and Gloucestershire Cancer Alliance (SWAG), who are promoting the use of innovations to help achieve the NHS Long Term Plan and cancer recovery.
Further real-world validation of the red dot® CXR product will take place over the next year through a multi-site randomised control trial, as part of Behold.ai’s successful award of a Phase 4 NHSX AI Award. The goal of this study will be to generate strong evidence for the national adoption of the red dot® CXR product, including benefits of its use in terms of patient outcomes, cost and time savings. Interim results for this study are expected to be available by January 2023.
References
- Dyer, T., Dillard, L., Harrison, M., Morgan, T. N., Tappouni, R., Malik, Q., & Rasalingham, S. (2021). Diagnosis of normal chest radiographs using an autonomous deep-learning algorithm. Clinical Radiology, 76(6), 473-e9.
Interested in talking to us about AI?
Get in touch to learn more about how red dot® can make a different to your patients and your organisation.